Prof. David A. Lowther
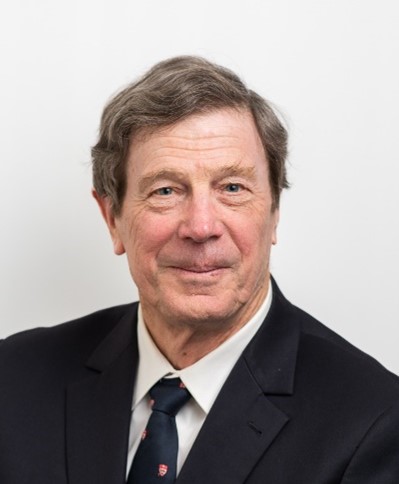
David Lowther received his Ph.D. degree in 1973 from Brighton Polytechnic in the United Kingdom before moving to Imperial College, London where he spent 6 years in a post-doctoral position. In 1979 he moved to McGill University in Montreal, Canada as an Associate Professor in the Department of Electrical Engineering and was promoted to Full Professor in 1986. His research relates to the design and analysis of low frequency electromagnetic devices, including applications of artificial intelligence. In 1978, he co-founded Infolytica Corporation which developed computer aided design tools for low frequency systems. The company was acquired by Siemens DISW in 2017 and he is currently a Technical Director in Technology and Innovation at Siemens. He has published over 400 conference and journal papers and is a Fellow of the Institution of Engineering Technology, the Institute of Electrical and Electronic Engineers and the Canadian Academy of Engineering.
Presentation: Machine learning based digital twins in the design of electromagnetic devices
Designing electromagnetic devices requires models of the performance of candidate solutions. It is an iterative process which gradually increases in complexity with the final model, ideally, being a full multi-physics digital twin. Making design decisions requires information which allows competing designs to be evaluated as well as ensuring that the final system meets all the user requirements. The earlier design decisions are made, the more effective the design process. Complete, traditional, numerical models are both expensive to construct and to analyze, although, usually, significantly cheaper than physical prototypes. Generative machine learning systems have demonstrated the potential to increase the amount of information at the start– effectively “front-loading” the design process. In turn, this can significantly reduce the time needed to create a final design. The presentation will address the application of both machine learning based digital twins as well as more conventional numerical approaches.