Prof. Yongjian Li
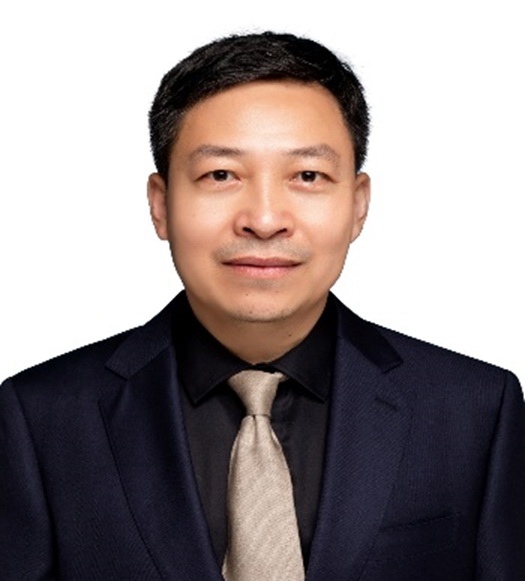
Yongjian Li, Ph.D. is a Professor at the School of Electrical Engineering, Hebei University of Technology, renowned for his expertise in electromagnetic analysis and simulation of high-power electrical systems. With a career dedicated to advancing electrical engineering research, he has led over 20 major national projects, including key initiatives under the National Natural Science Foundation of China and national key R&D programs. His scholarly contributions encompass 100+ SCI publications, three monographs, and 30 authorized invention patents, three of which have achieved commercial success. Recognized for his groundbreaking work, Dr. Li has been awarded the First Prize for Scientific and Technological Progress by Hebei Province and the Chinese Society of Electrotechnical Technology, alongside honors such as the Hebei Outstanding Youth Fund, Hebei Provincial Outstanding Expert, and Tianjin Youth Science and Technology Award. He serves as Director and Vice Chairman of the Professional Committee, China Electrotechnical Society, Director at the Hebei Key Laboratory of Flexible DC Transmission Equipment and Technology, and Vice Chairman of the Hebei Society of Electrical Engineers.
The main research interests are: Deep Belief Networks; Multi-Hysteresis Model Fusion; Three-Dimensional Spatial Hysteresis Model.
Presentation: Hysteresis modelling methods of soft magnetic materials based on deep neural network
Hysteresis modelling is a fundamental and hot issue in the field of electrical engineering. Traditional hysteresis models are usually fail to balance computational accuracy and generality. While deep neural networks (DNNs) have shown significant advantages in hysteresis models in handling complex nonlinear relationships, high-dimensional data, and dynamic characteristics.
In this report, deep neural networks are integrated into hysteresis modeling for magnetic materials, including forward and inverse models, as well as scalar and vector models. Based on these newly established models, the magnetization behavior of magnetic materials can be described more accurately, addressing the complexity of inverse problems and enabling more accurate simulation of complex three-dimensional magnetization phenomena. Compared to traditional models, the new models offer improvements in both computational accuracy and efficiency.